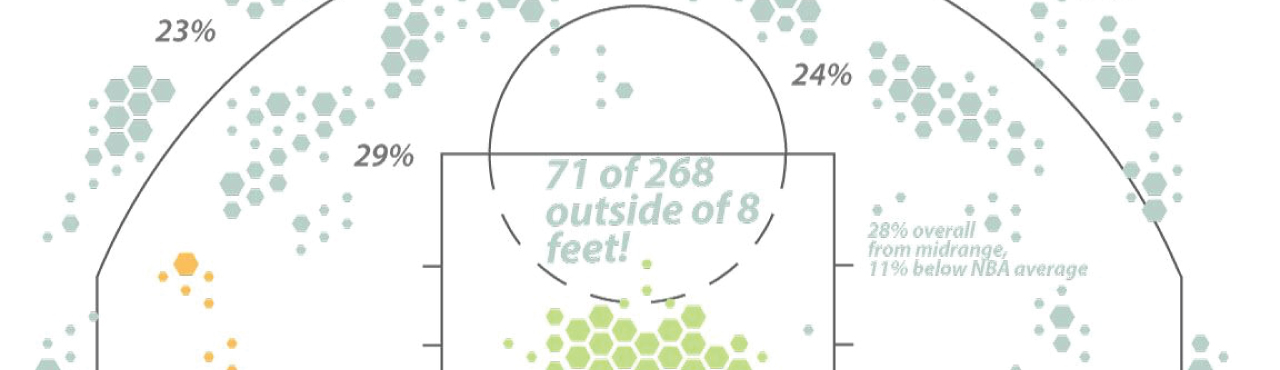
Program
Location: Ballroom A
9:00-9:10
Intro
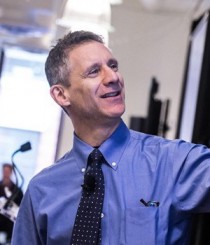
Andrew Gelman
Keynote: Uncovering Principles of Statistical Visualization
Andrew Gelman, Department of Statistics and Department of Political Science, Columbia University
Abstract: Visualizations are central to good statistical workflow, but it has been difficult to establish general principles governing their use. We will try to back out some principles of visualization by considering examples of effective and ineffective uses of graphics in our own applied research. We consider connections between three goals of visualization: (a) vividly displaying results, (b) exploration of unexpected patterns in data, and (c) understanding fitted models.
Bio: Andrew Gelman is a professor of statistics and political science and director of the Applied Statistics Center at Columbia University. He has received the Outstanding Statistical Application award from the American Statistical Association, the award for best article published in the American Political Science Review, and the Council of Presidents of Statistical Societies award for outstanding contributions by a person under the age of 40. His books include Bayesian Data Analysis (with John Carlin, Hal Stern, David Dunson, Aki Vehtari, and Don Rubin), Teaching Statistics: A Bag of Tricks (with Deb Nolan), Data Analysis Using Regression and Multilevel/Hierarchical Models (with Jennifer Hill), Red State, Blue State, Rich State, Poor State: Why Americans Vote the Way They Do (with David Park, Boris Shor, and Jeronimo Cortina), and A Quantitative Tour of the Social Sciences (co-edited with Jeronimo Cortina).
Andrew has done research on a wide range of topics, including: why it is rational to vote; why campaign polls are so variable when elections are so predictable; why redistricting is good for democracy; reversals of death sentences; police stops in New York City, the statistical challenges of estimating small effects; the probability that your vote will be decisive; seats and votes in Congress; social network structure; arsenic in Bangladesh; radon in your basement; toxicology; medical imaging; and methods in surveys, experimental design, statistical inference, computation, and graphics.
10:10-10:30
Poster Preview
(Posters will be on display in the coffee breaks)
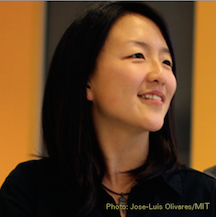
Been Kim
Keynote: Interpretability - now what?
Been Kim, Google
Abstract: In this talk, I hope to reflect on some of the progress made in the field of interpretable machine learning. We will reflect on where we are going as a field, and what are the things we need to be aware and be careful as we make progress. With that perspective, I will then discuss some of my recent work 1) sanity checking popular methods and 2) developing more lay person-friendly interpretability method.
Bio: Been Kim is a senior research scientist at Google Brain. Her research focuses on building interpretable machine learning - making ML understandable by humans for more responsible AI. The vision of her research is to make humans empowered by machine learning, not overwhelmed by it. She gave ICML tutorial on the topic in 2017, CVPR and MLSS at University of Toronto in 2018. She is a co-workshop Chair ICLR 2019, and has been an area chair at NIPS, ICML, AISTATS and FAT* conferences. In 2018, she gave a talk at G20 meeting on digital economy summit in Argentina. In 2019, her work called TCAV received UNESCO Netexplo award for "breakthrough digital innovations with the potential of profound and lasting impact on the digital society”. This work was also a part of CEO’s keynote at Google I/O 19'. She received her PhD. from MIT.
11:50-12:20
Paper Session: Application I
Albireo: An Interactive Tool for Visually Summarizing Computational Notebook Structure
John Wenskovitch, Jian Zhao, Scott Carter, Matthew Cooper, Chris North
Abstract: Computational notebooks have become a major medium for data exploration and insight communication in data science. Although expressive, dynamic, and flexible, in practice they are loose collections of scripts, charts, and tables that rarely tell a story or clearly represent the analysis process. This leads to a number of usability issues, particularly in the comprehension and exploration of notebooks. In this work, we design, implement, and evaluate Albireo, a visualization approach to summarize the structure of notebooks, with the goal of supporting more effective exploration and communication by displaying the dependencies and relationships between the cells of a notebook using a dynamic graph structure. We evaluate the system via a case study and expert interviews, with our results indicating that such a visualization is useful for an analyst’s self-reflection during exploratory programming, and also effective for communication of narratives and collaboration between analysts.
[Best Paper] PeckVis: A Visual Analytics Tool to Analyze Dominance Hierarchies in Small Groups (Accepted by TVCG)
Darius Coelho, Ivan Chase, Klaus Mueller
Abstract: The formation of social groups is defined by the interactions among the group members. Studying this group formation process can be useful in understanding the status of members, decision-making behaviors, spread of knowledge and diseases, and much more. A defining characteristic of these groups is the pecking order or hierarchy the members form which help groups work towards their goals. One area of social science deals with understanding the formation and maintenance of these hierarchies, and in our work, we provide social scientists with a visual analytics tool - PeckVis - to aid this process. While online social groups or social networks have been studied deeply and lead to a variety of analyses and visualization tools, the study of smaller groups in the field of social science lacks the support of suitable tools. Domain experts believe that visualizing their data can save them time as well as reveal findings they may have failed to observe. We worked alongside domain experts to build an interactive visual analytics system to investigate social hierarchies. Our system can discover patterns and relationships between the members of a group as well as compare different groups. The results are presented to the user in the form of an interactive visual analytics dashboard. We demonstrate that domain experts were able to effectively use our tool to analyze animal behavior data. While the primary application of PeckVis is to animal interaction data, we also demonstrate how other interaction-based data, such as a debate, can be effectively transformed and analyzed with our tool.
2:20-2:50
Paper Sesssion: Application II
DELFI: Mislabelled Human Context Detection Using Multi-Feature Similarity Linking
Hamid Mansoor, Walter Gerych, Luke Buquicchio, Kavin Chandrasekaran, Elke Rundensteiner, Emmanuel O Agu
Abstract: Context Aware (CA) systems that model and adapt to the behaviors of their users have many real-world applications. CA systems generally require accurately labeled training data in order to learn parameters necessary to model users' context behavior. Unfortunately, it is challenging to gather sufficient realistic context data in a controlled study environment where labels can be reliably gathered. For this reason, recent studies have made use of in-the-wild context data, where data is gathered through some passive sensing device such as a smartphone while users are periodically required to supply their context labels. However context labels gathered through in-the-wild studies are not completely reliable as users are error prone and may provide incomplete or inaccurate labels for their data, which in turn makes it difficult to build robust CA models. We propose a visual analytics approach to finding instances of mislabelled data and cleaning human context data. Data visualizations enable analysts to highlight similar data to find patterns and anomalies in human behaviors. However, such an approach is challenging when working with erroneous human-labelled data as linking data based on similar context labels is flawed as the labels themselves are in question. To that end, we present DELFI, a visual analytics framework for identifying and relabeling mislabeled and unlabeled instances of user-labeled human context data. DELFI visually identifies likely-mislabeled instances by color-coding an anomaly score. Additionally, DELFI links instances based on a novel concept called Multi-Feature Similarity Linking, which helps to identify the likely true labels of mislabeled and unlabeled instances. We demonstrate the utility of our approach with detailed use cases and evaluation from domain experts.
A Visual Analytics Framework for Analyzing Parallel and Distributed Computing Applications
Kelvin Li, Takanori Fujiwara, Suraj Padmanaban Kesavan, Caitlin Ross, Misbah Mubarak, Christopher Carothers, Robert Ross, Kwan-Liu Ma
Abstract: To optimize the performance and efficiency of HPC applications, programmers and analysts often need to collect various performance metrics for each computer at different time points as well as the communication data between the computers. This results in a complex dataset that consists of multivariate time-series and communication network data, thereby makes debugging and performance tuning of HPC applications challenging. Automated analytical methods based on statistical analysis and unsupervised learning are often insufficient to support such tasks without the background knowledge from the application programmers. To better explore and analyze a wide spectrum of HPC datasets, effective visual data analytics techniques are needed. In this paper, we present a visual analytics framework for analyzing HPC datasets produced by parallel discrete-event simulations (PDES). Our framework leverages automated time-series analysis methods and effective visualizations to analyze both multivariate time-series and communication network data. Through several case studies for analyzing the performance of PDES, we show that our visual analytics techniques and system can be effective in reasoning multiple performance metrics, temporal behaviors of the simulation, and the communication patterns.
2:50-3:35
Paper Session: Encoding
Outliagnostics: Visualizing Temporal Discrepancy in Outlying Signatures of Data Entries
Vung Pham, Tommy Dang
Abstract: This paper presents an approach to analyzing orthogonal pairwise projections focusing on identifying observations that are significant in calculating the outliers of a scatterplot. We also propose a prototype, called Outliagnostics, to guide users when interactively exploring abnormalities in large time series. Instead of focusing on detecting outliers at each time point, we monitor and display the temporal discrepant signatures of each data entry concerning the overall distributions. Our prototype is designed to handle the types of doubly-multivariate data series in parallel. To highlight the benefits and performance of our approach, we illustrate and validate the use of Outliagnostics on real-world datasets of various sizes in different parallelism configurations.
Pollux: Interactive Cluster-First Projections of High-Dimensional Data
John Wenskovitch, Chris North
Abstract: Semantic interaction is an technique relying upon the interactive semantic exploration of data. While manipulating data items within a visualization, an underlying model learns from the intent underlying these interactions and updates the parameters of the model controlling the visualization. In this work, we propose, implement, and evaluate a model which defines clusters within this data projection, then projects these clusters into a two-dimensional space using a “proximity∼similarity” metaphor. These clusters act as targets against which data values can be manipulated, providing explicit user-driven cluster membership assignments to train the underlying models. Using this cluster-first approach can improve the speed and efficiency of laying out a projection of high-dimensional data, with the tradeoff of distorting the global projection space.
[Best Paper Runner Up] Glyphboard: Visual Exploration of High-dimensional Data Combining Glyphs with Dimensionality Reduction (Accepted by TVCG)
Dietrich Kammer, Mandy Keck, Thomas Gründer, Alexander Maasch, Thomas Thom, Martin Kleinsteuber, Rainer Groh
Abstract: Rigorous data science is interdisciplinary at its core. In order to make sense of high-dimensional data, data scientists need to enter into a dialogue with domain experts. We present Glyphboard, a visualization tool that aims to support this dialogue. Glyphboard is a zoomable user interface that combines well-known methods such as dimensionality reduction and glyph-based visualizations in a novel, seamless, and immersive tool. While the dimensionality reduction affords a quick overview over the data, glyph-based visualizations are able to show the most relevant dimensions in the data set at one glance. We contribute an open-source prototype of Glyphboard, a general exchange format for high-dimensional data, and a case study with nine data scientists and domain experts from four exemplary domains in order to evaluate how the different visualization and interaction features of Glyphboard are used.
4:10-4:40
Paper Session: Perception
Sherpa: Leveraging User Attention for Computational Steering in Visual Analytics
Zhe Cui, Jayaram Kancherla, Héctor Corrada, Niklas Elmqvist
Abstract: We present Sherpa, a computational steering mechanism for progressive visual analytics that automatically prioritizes computations performed on the dataset based on the analyst's navigational behavior in the data. The intuition is that navigation in data space is an indication of the analyst's attention and thus interest in the data. Our example web-based client/server implementation of Sherpa provides computational modules for genomic data analysis, where independent computations calculate test statistics relevant to biological inferences about gene regulation in various tumor types and their corresponding normal tissues. The position and dimension of the navigation window on the genomic sequence over time is used to prioritize these computations to genomic regions favored by the user. In a study with experienced genomic and visualization analysts, we found that Sherpa provided comparable accuracy to the offline condition, where all computations were completed prior to analysis, while enabling shorter completion times. We also provide a second illustrative example on large-scale stock market analysis over time (Sherpa Stock).
Task-Oriented Optimal Sequencing of Visualization Charts
Danqing Shi, Yang Shi, Xinyue Xu, Nan Chen, Siwei Fu, Hongjin Wu, Nan Cao
Abstract: A chart sequence is used to describe a series of visualization charts generated in the exploratory analysis by data analysts. It provides not only information details in each chart but also a logical relationship among charts, which assists in interpreting the exploratory process, helping analysts understand the data and make the following decisions. We present a novel chart sequencing method based on reinforcement learning to capture the connections between charts in the context of analysis tasks. The proposed method formulates a chart sequencing procedure as an optimization problem, which seeks an optimal policy to sequencing charts for the specific analysis task. In our method, a novel reward function is introduced, which takes both the analysis task and the factor of human cognition into consideration. We evaluate our method under the application scenarios of visualization recommendation, sequencing charts for reasoning analysis results, and making a chart design choice.
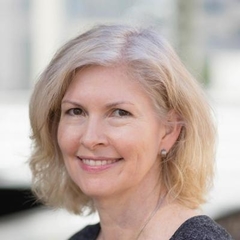
Jenny Bryan
Keynote: Behind every great vis ... there's a great deal of wrangling
Jenny Bryan, RStudio
Abstract: If you are struggling to make a plot, tear yourself away from stackoverflow for a moment and ... take a hard look at your data. Is it really in the most favorable form for the task at hand? I must confess that I am no visualization pro. But even as a data analyst, I've made a very great number of plots. Time and time again I have found that my visualization struggles are really a symptom of unfinished data wrangling. I will give an overview of the data wrangling landscape, with a special emphasis on R (my language of choice), but including high-level principles that are applicable to those working in other languages.
Bio: Jenny Bryan (twitter, GitHub) is a Software Engineer at RStudio, working on the team lead by Hadley Wickham. This team brings you the popular set of packages known as the Tidyverse, as well as a set of low-level infrastructure packages. She is on leave from being an Associate Professor of Statistics at the University of British Columbia. Jenny has an undergraduate degree in Economics and German Literature from Yale University and earned her PhD in Biostatistics at UC Berkeley.
Jenny has been using and teaching R (or S!) for 20 years, most recently in STAT 545 and Software Carpentry. Other aspects of her R life include ordinary membership in the R Foundation, work with rOpenSci, development/maintenance of R packages (such as readxl), and leading the curriculum development for UBC's Master of Data Science.