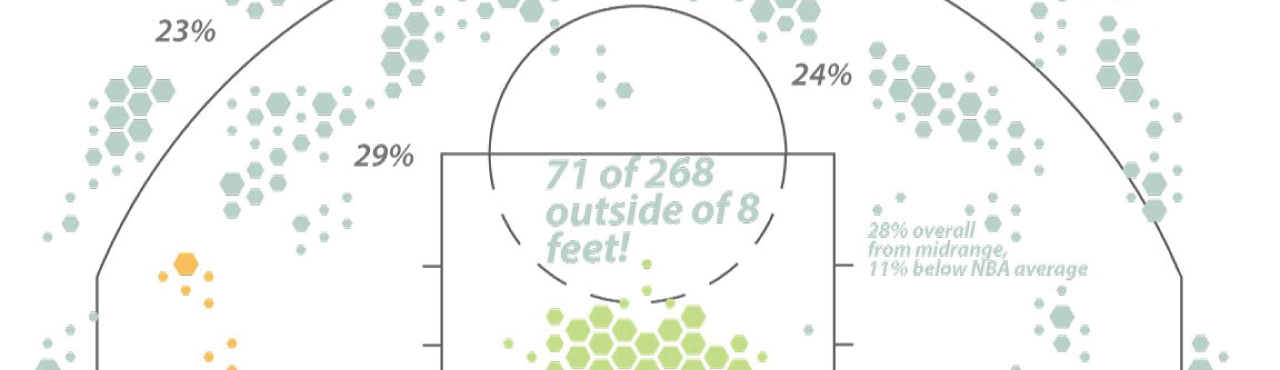
Program
VDS @IEEE VIS 2022
Registration at http://ieeevis.org/year/2022/info/registration/conference-registration .
Sun. Oct 16, 2022, 9am - 12pm (CDT), 2022
9:00am~9:05am CDT
9:05am~9:40am CDT
Opening
9:05am~9:40am CDT Keynote
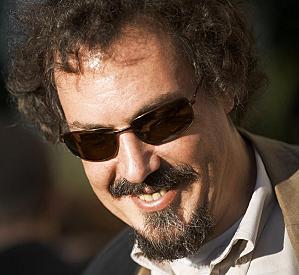
Jean-Daniel Fekete
Keynote: Scalability with Progressive Data Science
Jean-Daniel Fekete, Université Paris-Saclay and Inria
Abstract: Data science is making progress every day, thanks to the fast evolution of all its parts, such as databases, machine learning, simulation, and visualization. However, when it comes to the exploration of data resulting from analytical computations, I will explain why scalability remains an issue. The standard method for addressing scalability consists of adding more resources: more processors, more GPUs, more memory, and faster networks. Unfortunately, this method will not solve the scalability problem alone, because it does not solve the crucial issues of maintaining latency under critical limits to allow exploration, and of taming human attention during long-lasting computations.
Data Science uses ad-hoc methods to try to address scalability, but I will show that they remain unsatisfactory.
Progressive Data Analysis (PDA) emerged about a decade ago to address this scalability problem, showing promising solutions. I will demonstrate a few examples, such as the exploration of patient pathways at scale and high-dimensional data analysis. However, PDA is still lagging behind, and I will argue, this is mainly due to the domain boundaries coming from academic research. A roadmap is, therefore, necessary to progress towards a unified solution crossing these boundaries.
Bio: Jean-Daniel Fekete is a Senior Research Scientist at Inria, France, head of the Research Lab Aviz at Université Paris-Saclay and Inria. He received his PhD in Computer Science in 1996 from Université Paris-Sud (now Université Paris-Saclay). He was recruited by Inria in 2002 and became a Senior Research Scientist in 2006. His main research areas are Visual Analytics, Information Visualization, and Human-Computer Interaction. He published more than 150 articles in international conferences and journals, including the most prestigious in visualization (TVCG, InfoVis, EuroVis, PacificVis) and Human-Computer Interaction (CHI, UIST).
He has been granted the IEEE VGTC Visualization Career Award 2020 and is a member of the IEEE VGTC Visualization Academy, and ACM SIGCHI Academy. He is a member of the Eurographics publication board, and Associate Editor in Chief of IEEE Transactions on Visualization and Computer Graphics. Jean-Daniel Fekete was the Chair of the EuroVis Best PhD Award Committee 2017-2021, the General Chair of the IEEE VIS Conference in 2014, the first time it was held outside of the USA in Paris, and the President of the French-Speaking HCI Association (AFIHM) 2009-2013.
09:40am~10:25am CDT
Paper Session
9:41am~9:52pm CDT
Yuncong Yu, Dylan Kruyff, Jiao Jiao, Tim Becker and Michael Behrisch
Abstract: We present PSEUDo, a visual pattern retrieval tool for multivariate time series. It aims to overcome the uneconomic (re-)training problem accompanying deep learning-based methods. Very high-dimensional time series emerge on an unprecedented scale due to increasing sensor usage and data storage. Visual pattern search is one of the most frequent tasks on time series. Automatic pattern retrieval methods often suffer from inefficient training data, a lack of ground truth labels, and a discrepancy between the similarity perceived by the algorithm and required by the user or the task. Our proposal is based on the query-aware locality-sensitive hashing technique to create a representation of multivariate time series windows. It features sub-linear training and inference time with respect to data dimensions. This performance gain allows an instantaneous relevance-feedback-driven adaption to converge to users’ similarity notion. We demonstrate PSEUDo’s performance in terms of accuracy, speed, steerability, and usability through quantitative benchmarks with representative time series retrieval methods and a case study. We find that PSEUDo detects patterns in high-dimensional time series efficiently, improves the result with relevance feedback through feature selection, and allows an understandable as well as user-friendly retrieval process.
9:52am~10:03pm CDT
Eren Cakmak, Johannes Fuchs, Dominik Jäckle, Tobias Schreck, Ulrik Brandes, Daniel Keim
Abstract: Many data analysis problems rely on dynamic networks, such as social or communication network analyses. Providing a scalable overview of long sequences of such dynamic networks remains challenging due to the underlying large-scale data containing elusive topological changes. We propose two complementary pixel-based visualizations, which reflect occurrences of selected sub-networks (motifs) and provide a time-scalable overview of dynamic networks: a network-level census (motif significance profiles) linked with a node-level sub-network metric (graphlet degree vectors) views to reveal structural changes, trends, states, and outliers. The network census captures significantly occurring motifs compared to their expected occurrences in random networks and exposes structural changes in a dynamic network. The sub-network metrics display the local topological neighborhood of a node in a single network belonging to the dynamic network. The linked pixel-based visualizations allow exploring motifs in different-sized networks to analyze the changing structures within and across dynamic networks, for instance, to visually analyze the shape and rate of changes in the network topology. We describe the identification of visual patterns, also considering different reordering strategies to emphasize visual patterns. We demonstrate the approach's usefulness by a use case analysis based on real-world large-scale dynamic networks, such as the evolving social networks of Reddit or Facebook.
10:03am~10:14pm CDT
Yuren Pang, Ruotong Wang, Joely Nelson, Leilani Battle
Abstract: Data science workers increasingly collaborate on large-scale projects before communicating insights to a broader audience in the form of visualization. While prior work has modeled how data science teams, oftentimes with distinct roles and work processes, communicate knowledge to outside stakeholders, we have little knowledge of how data science workers communicate intermediately before delivering the final products. In this work, we contribute a nuanced description of the intermediate communication process within data science teams. By analyzing interview data with 8 self-identified data science workers, we characterized the data science intermediate communication process with four factors, including the types of audience, communication goals, shared artifacts, and mode of communication. We also identified three overarching challenges in the current communication process. We also discussed design implications that might inform better tools that facilitate intermediate communication within data science teams.
10:14am~10:25pm CDT
Agapi Rissaki, Bruno Scarone, David Liu, Aditeya Pandey, Brennan Klein, Tina Eliassi-Rad, Michelle A. Borkin
Abstract: The issue of bias (i.e., systematic unfairness) in machine learning models has recently attracted the attention of both researchers and practitioners. For the graph mining community in particular, an important goal toward algorithmic fairness is to detect and mitigate bias incorporated into graph embeddings since they are commonly used in human-centered applications, e.g., social-media recommendations. However, simple analytical methods for detecting bias typically involve aggregate statistics which do not reveal the sources of unfairness. Instead, visual methods can provide a holistic fairness characterization of graph embeddings and help uncover the causes of observed bias. In this work, we present BiaScope, an interactive visualization tool that supports end-to-end visual unfairness diagnosis for graph embeddings. The tool is the product of a design study in collaboration with domain experts. It allows the user to (i) visually compare two embeddings with respect to fairness, (ii) locate nodes or graph communities that are unfairly embedded, and (iii) understand the source of bias by interactively linking the relevant embedding subspace with the corresponding graph topology. Experts' feedback confirms that our tool is effective at detecting and diagnosing unfairness. Thus, we envision our tool both as a companion for researchers in designing their algorithms as well as a guide for practitioners who use off-the-shelf graph embeddings.
10:25am-10:55am CDT
Break
10:55am-12:15am CDT
Paper Session 2
10:55am~11:03am CDT
Comparison of Computational Notebook Systems for Interactive Visual Analytics
Han Liu
Abstract: Existing notebook platforms have different capabilities for supporting visual analytics use. It is not clear which platform to choose for implementing visual analytics notebooks. In this work, we investigated the problem using Andromeda, an interactive dimension reduction algorithm, and implemented it using three different notebook platforms: 1) Python-based Jupyter Notebook, 2) JavaScript-based Observable Notebook, and 3) Jupyter Notebook embedding both Python (data science use) and JavaScript (visual analytics use). We also made comparisons for all the notebook platforms via a case study based on metrics such as programming difficulty, notebook organization, interactive performance, and UI design choice. Furthermore, guidelines are provided for data scientists to choose one notebook platform for implementing their visual analytics notebooks in various situations. Laying the groundwork for future developers, advice is also given on architecting better notebook platforms.
11:03am~11:14am CDT
Interactive Visualization for Data Science Scripts
Rebecca Faust, Carlos Scheidegger, Katherine E. Isaacs, William Bernstein, Michael Sharp, Chris North
Abstract: As the field of data science continues to grow, so does the need for adequate tools to understand and debug data science scripts. Current debugging practices fall short when applied to a data science setting, due to the exploratory and iterative nature of analysis scripts. Additionally, computational notebooks, the preferred scripting environment of many data scientists, present additional challenges to understanding and debugging workflows, including the non-linear execution of code snippets. This paper presents Anteater, a trace-based visual debugging method for data science scripts. Anteater automatically traces and visualizes execution data with minimal analysts input. These visualizations illustrate execution and value behaviors that assist in understanding the results of analysis scripts. To maximize the number of workflows supported, we present prototype implementations in both Python and Jupyter. Last, to demonstrate Anteater’s support for analysis understanding tasks, we provide two usage scenarios on real world analysis scripts.
11:14am~11:25am CDT
Maximilian T. Fischer, Frederik L. Dennig, Daniel Seebacher, Daniel Keim, Mennatallah El-Assady
Abstract: The automated analysis of digital human communication data often focuses on specific aspects such as content or network structure in isolation. This can provide limited perspectives while making cross-methodological analyses in domains like investigative journalism difficult. Communication research in psychology and the digital humanities instead stresses the importance of a holistic approach to overcome these limiting factors. In this work, we conduct an extensive survey on the properties of over forty semi-automated communication analysis systems and investigate how they cover concepts described in theoretical communication research. From these investigations, we derive a design space and contribute a conceptual framework based on communication research, technical considerations, and the surveyed approaches. The framework describes the systems' properties, capabilities, and composition through a wide range of criteria organized in the dimensions (1) Data, (2) Processing and Models, (3) Visual Interface, and (4) Knowledge Generation. These criteria enable a formalization of digital communication analysis through visual analytics, which, we argue, is uniquely suited for this task by tackling automation complexity while leveraging domain knowledge. With our framework, we identify shortcomings and research challenges, such as group communication dynamics, trust and privacy considerations, and holistic approaches. Simultaneously, our framework supports the evaluation of systems.
11:25am~11:55am CDT
11:25am~11:55am CDT
Keynote 2
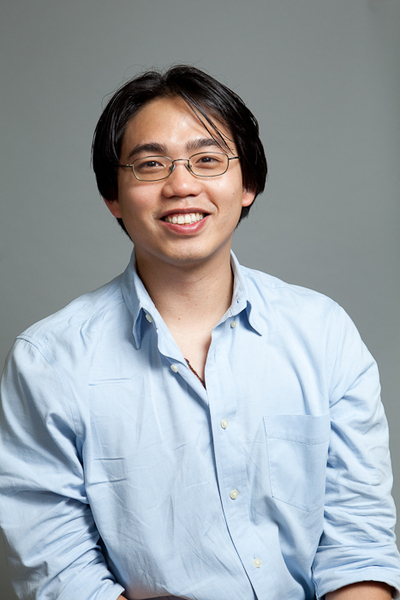
Remco Chang
Keynote: Towards a Unifying Theory of Data, Task, and Visualization with a Grammar of Hypothesis
Remco Chang, Tufts University
Abstract: In this talk, I present our recent work on developing a unifying theory that encompasses data, visualization, and analysis (tasks) based on a grammar of a (scientific) hypotheses. The grammar provides a mechanism to consider data, task, and visualization as “hypothesis spaces.” A “data hypothesis space” is a space of all the hypotheses that a dataset can be used to answer, a “visualization hypothesis space” is the space of hypotheses that a visualization can be used to validate, and an “analyst hypothesis space” is the space of the hypotheses that an analyst would like the answer to. With the hypothesis grammar, we can examine the relations between the three spaces and their practical implications.
In addition, with the formalization of a grammar, we can reconsider some classic research topics central to visualization research. For example, visualization recommendation can be thought of as finding a visualization that maximizes the intersection between the visualization hypothesis space and the others. Evaluating a visual analytics system can be thought of as evaluating the system’s capability to support a user in exploring a data hypothesis space. I will present the foundation of our grammar and introduce some promising new research directions that may become possible with our proposed formalization.
Bio: Remco Chang is an Associate Professor in the Computer Science Department at Tufts University. He received his BA from Johns Hopkins University in 1997 in Computer Science and Economics, MSc from Brown University in 2000, and PhD in Computer Science from UNC Charlotte in 2009. Prior to his PhD, he worked for Boeing developing real-time flight tracking and visualization software, followed by a position at UNC Charlotte as a research scientist. His current research interests include visual analytics, information visualization, HCI, and databases. His research has been funded by the NSF, DARPA, the Walmart Foundation, Army, Navy, DHS, MIT Lincoln Lab, and Draper. He has had best paper, best poster, and honorable mention awards at InfoVis, VAST, CHI, and VDA. He is currently an associate editor for the ACM TiiS, and he is the papers chair for the IEEE Visual Analytics conference (VAST) in 2018 and 2019. He received the NSF CAREER Award in 2015. He has supervised 3 PhD students, co-supervised 5 PhD students, and mentored 3 postdoctoral researchers, some of whom became professors in Computer Science at Smith College, DePaul University, Washington University in Saint Louis, University of Maryland, the University of San Francisco, Bucknell University, San Francisco State University, and the University of Utrecht (Netherlands).
VDS @ KDD 2022 (Past)
Conference registration at https://www.kdd.org/kdd2022/registration.html.
Sun. Aug 14, 2022, 1pm - 4:10pm (CDT)
1:00pm~1:10pm CDT
1:10pm~2:10pm CDT
Opening
1:10pm~2:10pm CDT Keynote
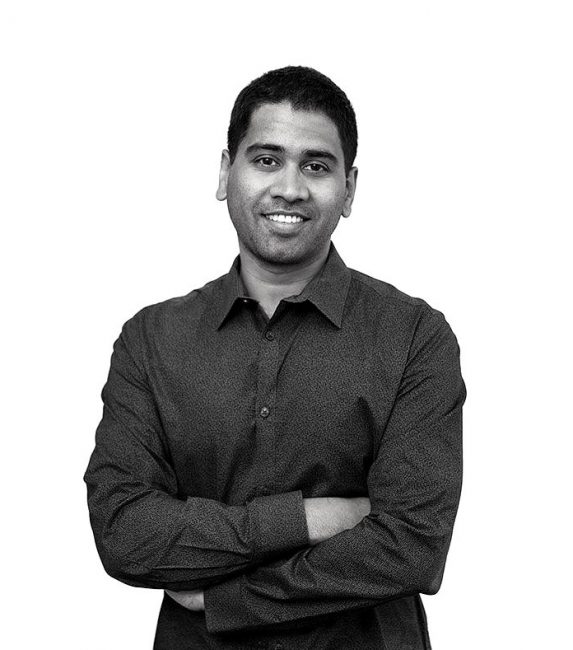
Kalyan Veeramachaneni
Keynote: Towards Usable Machine Learning
Kalyan Veeramachaneni, Massachusetts Institute of Technology
Abstract: TBD.
Recording: link. Password: 9DR=w2D*
Recording: link. Password: 9DR=w2D*
Bio: Dr. Kalyan Veeramachaneni is a Principal Research Scientist at the Laboratory for Information and Decision System (LIDS) at MIT. He directs a research group called Data to AI in the new MIT Schwarzman College of Computing. His group focuses on building large-scale AI systems that work alongside humans, continuously learning from data, generating predictions and integrating those predictions into human decision-making. The group develops foundational algorithms, abstractions, and systems to enable these three tasks at scale. Algorithms, systems and open-source software developed by the group are deployed for applications in the financial, medical, and education sectors. Kalyan was the co-founder of PatternEx (acq. by Corelight), a cybersecurity company that adapts machine learning models based on real-time analyst feedback. He was also the co-founder of FeatureLabs (acq. by Alteryx), a data science automation company. He is currently a co-founder of DataCebo which focuses on improving data access and availability through synthetic data generation. Kalyan has published over 70 publications and his work on AI-driven solutions for data science and cybersecurity has been covered by major media outlets, including the Washington Post, CBS News, Wired, Forbes and Newsweek. He received his Masters in Computer Engineering and Ph.D in Electrical Engineering in 2009, both from Syracuse University. He joined MIT in 2009.
2:10pm~2:20pm
Break
2:20pm~3:00pm CDT
Paper Session
2:20pm~2:40pm CDT
Yuncong Yu, Dylan Kruyff, Jiao Jiao, Tim Becker and Michael Behrisch
Abstract: We present PSEUDo, a visual pattern retrieval tool for multivariate time series. It aims to overcome the uneconomic (re-)training problem accompanying deep learning-based methods. Very high-dimensional time series emerge on an unprecedented scale due to increasing sensor usage and data storage. Visual pattern search is one of the most frequent tasks on time series. Automatic pattern retrieval methods often suffer from inefficient training data, a lack of ground truth labels, and a discrepancy between the similarity perceived by the algorithm and required by the user or the task. Our proposal is based on the query-aware locality-sensitive hashing technique to create a representation of multivariate time series windows. It features sub-linear training and inference time with respect to data dimensions. This performance gain allows an instantaneous relevance-feedback-driven adaption to converge to users’ similarity notion. We demonstrate PSEUDo’s performance in terms of accuracy, speed, steerability, and usability through quantitative benchmarks with representative time series retrieval methods and a case study. We find that PSEUDo detects patterns in high-dimensional time series efficiently, improves the result with relevance feedback through feature selection, and allows an understandable as well as user-friendly retrieval process.
2:40pm~3:00pm CDT
Wei Han, Yangqiming Wang, Christian Boehm and Junming Shao
Abstract: Although deep neural networks have shown well-performance in various tasks, the poor interpretability of the models is always criticized. In the paper, we propose a new interpretable neural network method, by embedding neurons into the semantic space to extract their intrinsic global semantics. In contrast to previous methods that probe latent knowledge inside the model, the proposed semantic vector externalizes the latent knowledge to static knowledge, which is easy to exploit. Specifically, we assume that neurons with similar activation are of similar semantic information. Afterwards, semantic vectors are optimized by continuously aligning activation similarity and semantic vector similarity during the training of the neural network. The visualization of semantic vectors allows for a qualitative explanation of the neural network. Moreover, we assess the static knowledge quantitatively by knowledge distillation tasks. Empirical experiments of visualization show that semantic vectors describe neuron activation semantics well. Without the sample-by-sample guidance from the teacher model, static knowledge distillation exhibit comparable or even superior performance with existing relation-based knowledge distillation methods.
3:00pm~3:10pm CDT
Break
3:10pm~4:10pm CDT
3:10pm~4:10pm CDT
Closing Keynote
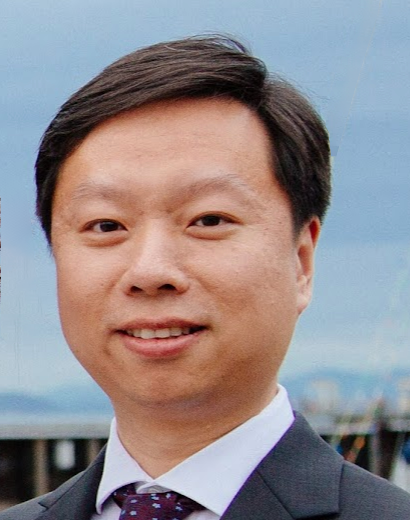
Leo Zhicheng Liu
Keynote: Towards Scalable and Interpretable Visual Analytics
Leo Zhicheng Liu, University of Maryland College Park
Abstract: Knowledge discovery on large-scale complex data is challenging. Not only do we need to devise efficient methods to extract insights, we must also enable users to interpret, trust and incorporate their domain knowledge into the automated results. How do we combine data mining, machine learning, and interactive visualization to address this problem? In this talk, I will review related research projects in the context of exploring, summarizing, and modelling temporal event sequence data for various application domains. Through our investigation, we identify symbiotic relationships between automated algorithms and visualizations: data mining and machine learning techniques suggest salient patterns and predictions to visualize; visualizations, on the other hand, can support data analysis across multiple levels of granularity, uncover potential limitations in automated approaches, and inspire new algorithms and techniques. Reflecting upon past experiences, I will discuss challenges and opportunities in tightly coupling automated algorithms with interactive visual interfaces for effective knowledge discovery.
Recording: link. Password: 9DR=w2D*
Recording: link. Password: 9DR=w2D*
Bio: Dr. Zhicheng Liu is an assistant professor in the department of computer science at University of Maryland. His research focuses on scalable methods to represent and interact with complex data, as well as techniques and systems to support the design and authoring of expressive data visualizations. Before joining UMD, he worked at Adobe Research as a research scientist and Stanford University as a postdoc fellow. He obtained his PhD at Georgia Tech. His work has been recognized with a Test-of-Time award at IEEE VIS, and multiple Best Paper Awards and Honorable Mentions at ACM CHI and IEEE VIS.