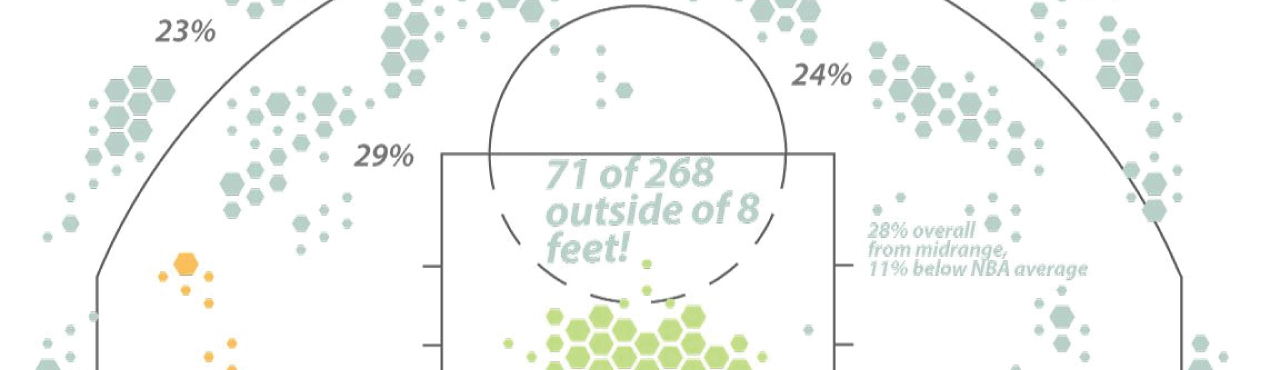
Program
VDS @IEEE VIS 2023
Registration at http://ieeevis.org/year/2023/info/registration/conference-registration .
Mon. Oct 23, 2023, 2:00 PM-5:00 PM AEDT (UTC+11)
2:00pm - 2:05pm
9:05am~9:40am CDT
Opening
2:05pm - 2:45pmKeynote 1: Dr Emi Tanaka
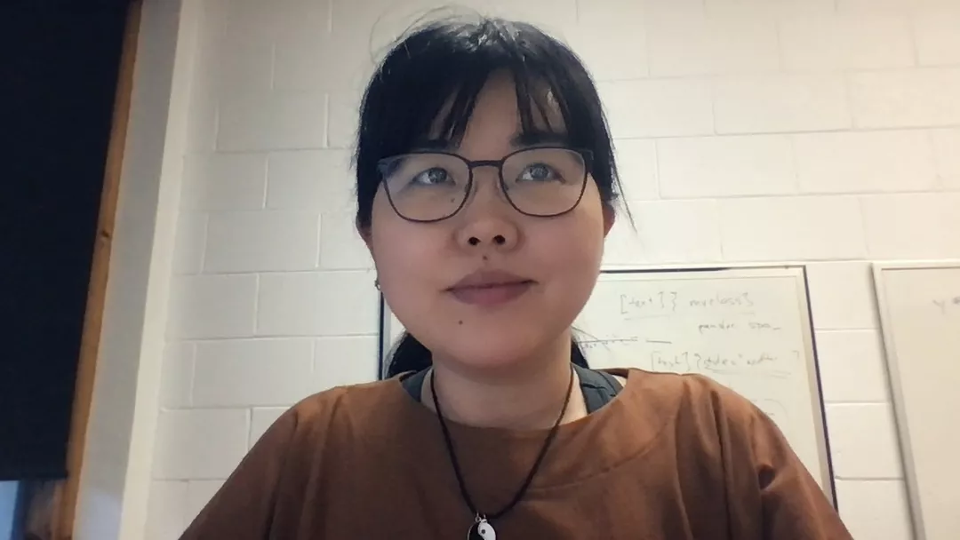
Emi Tanaka
Keynote: (Re)marrying statistical thinking and visualisations
Emi Tanaka, Australian National University
Abstract: Visualisation captivates one of our primal senses, i.e. the sight, but we need to engage our cognition to interpret its meaning. In that sense, we cannot, and should not, decouple statistical thinking from data visualisation, nor should we overlook the role of visualisation in statistical thinking. In this talk, I will share cases where reciprocal cooperation is beneficial to us and others around us.
Bio: Dr. Emi Tanaka is a Senior Lecturer in Statistics at the Biological Data Science Institute (and the Research School of Finance, Actuarial Studies and Statistics) at the Australian National University. Her primary interest is to develop impactful methods and tools that can be readily used by practitioners. She interfaces across multiple disciplines to bridge statistical concepts and findings to a broad range of individuals. To this end, she has developed numerous open-source tools, primarily as R-packages, and resources aimed at making statistical methods accessible to a diverse audience. Emi demonstrates a proactive approach to community development and education through her involvement in the branches of the Statistical Society of Australia (SSA) and other committees. She is the current Vice-President of the SSA Victoria & Tasmanian Branch. Her contributions are recognised with the SSA Distinguished Presenter's Award, SSA President’s Award for Leadership in Statistics, and being featured in the list of 60 prominent Australian statisticians in the Significance magazine.
02:45pm - 4:07pm
Paper Session
2:45pm - 2:56pm
[Best Paper] A Declarative Specification for Authoring Metrics Dashboards
Will Epperson, Kanit Wongsuphasawat, Allison Whilden, Fan Du, Justin Talbot
Abstract: Despite their ubiquity, authoring dashboards for metrics reporting in modern data analysis tools remains a manual, time-consuming process. Rather than focusing on interesting combinations of their data, users have to spend time creating each chart in a dashboard one by one. This makes dashboard creation slow and tedious. We conducted a review of production metrics dashboards and found that many dashboards contain a common structure: breaking down one or more metrics by different dimensions. In response, we developed a high-level specification for describing dashboards as sections of metrics repeated across the same dimensions and a graphical interface, Quick Dashboard, for authoring dashboards based on this specification. We present several usage examples that demonstrate the flexibility of this specification to create various kinds of dashboards and support a data-first approach to dashboard authoring
2:56pm - 3:07pm
Aardvark: Comparative Visualization of Data Analysis Scripts
Rebecca Faust, Carlos Scheidegger, Chris North
Abstract: Debugging programs is famously one of the most challenging aspects of programming. Data analysis scripts present additional challenges as debugging tasks are often more exploratory, such as comparing results under different parameter settings. In fact, a common exploratory debugging process is to run, modify, and re-run a script to observe the effects of the change. Analyst’s perform this process repeatedly as they explore different settings in their script. However, traditional debugging methods do not support direct comparison across script executions. To address this, we present Aardvark, a comparative trace-based debugging method for identifying and visualizing the differences between consecutive executions of analysis scripts. Aardvark traces two consecutive instances of a script, identifies the differences between them, and presents them through comparative visualizations. We present a prototype implementation in Python along with an extension to Jupyter notebooks and demonstrate Aardvark through two usage scenarios on real world analysis scripts.
3:15pm - 3:45pm
Break
3:45pm - 4:16pm
Paper Session 2
3:45pm - 4:56pm
Visual Comparison of Text Sequences Generated by Large Language Models
Rita Sevastjanova, Simon Vogelbacher, Andreas Spitz, Daniel Keim, Mennatallah El-Assady
Abstract: Causal language models have emerged as the leading technology for automating text generation tasks. Although these models tend to produce outputs that resemble human writing, they still suffer from quality issues (e.g., social biases). Researchers typically use automatic analysis methods to evaluate the model limitations, such as statistics on stereotypical words. Since different types of issues are embedded in the model parameters, the development of automated methods that capture all relevant aspects remains a challenge. To tackle this challenge, we propose a visual analytics approach that supports the exploratory analysis of text sequences generated by causal language models. Our approach enables users to specify starting prompts and effectively groups the resulting text sequences. To this end, we leverage a unified, ontology-driven embedding space, serving as a shared foundation for the thematic concepts present in the generated text sequences. Visual summaries provide insights into various levels of granularity within the generated data. Among others, we propose a novel comparison visualization that slices the embedding space and represents the differences between two prompt outputs in a radial layout. We demonstrate the effectiveness of our approach through case studies, showcasing its potential to reveal model biases and other quality issues.
4:56pm - 4:07pm
HPC ClusterScape: Increasing Transparency and Efficiency of Shared High-Performance Computing Clusters for Large-scale AI Models
Heungseok Park, Aeree Cho, Hyojun Jeon, Hayoung Lee, Youngil Yang, Sungjae Lee, Heungsub Lee, Jaegul Choo
Abstract: The emergence of large-scale AI models, like GPT-4, has significantly impacted academia and industry, driving the demand for high-performance computing (HPC) to accelerate workloads. To address this, we present HPCClusterScape, a visualization system that enhances the efficiency and transparency of shared HPC clusters for large-scale AI models. HPCClusterScape provides a comprehensive overview of system-level (e.g., partitions, hosts, and workload status) and application-level (e.g., identification of experiments and researchers) information, allowing HPC operators and machine learning researchers to monitor resource utilization and identify issues through customizable violation rules. The system includes diagnostic tools to investigate workload imbalances and synchronization bottlenecks in large-scale distributed deep learning experiments. Deployed in industrial-scale HPC clusters, HPCClusterScape incorporates user feedback and meets specific requirements. This paper outlines the challenges and prerequisites for efficient HPC operation, introduces the interactive visualization system, and highlights its contributions in addressing pain points and optimizing resource utilization in shared HPC clusters.
4:07pm - 4:16pm
NeighViz: Towards Better Understanding of Neighborhood Effects on Social Groups with Spatial Data
Yue Yu, Yifang Wang, Qisen Yang, Di Weng, Yongjun Zhang, Xiaogang Wu, Yingcai Wu, Huamin Qu
Abstract: Understanding how local environments influence individual behaviors, such as voting patterns or suicidal tendencies, is crucial in social science to reveal and reduce spatial disparities and promote social well-being. With the increasing availability of large-scale individual-level census data, new analytical opportunities arise for social scientists to explore human behaviors (e.g., political engagement) among social groups at a fine-grained level. However, traditional statistical methods mostly focus on global, aggregated spatial correlations, which are limited to understanding and comparing the impact of local environments (e.g., neighborhoods) on human behaviors among social groups. In this study, we introduce a new analytical framework for analyzing multi-variate neighborhood effects between social groups. We then propose NeighViz, an interactive visual analytics system that helps social scientists explore, understand, and verify the influence of neighborhood effects on human behaviors. Finally, we use a case study to illustrate the effectiveness and usability of our system.
4:16pm - 4:56pm
4:16pm - 4:56pm
4:56pm - 5:00pm
Keynote 2: Dr. Tamara Munzner
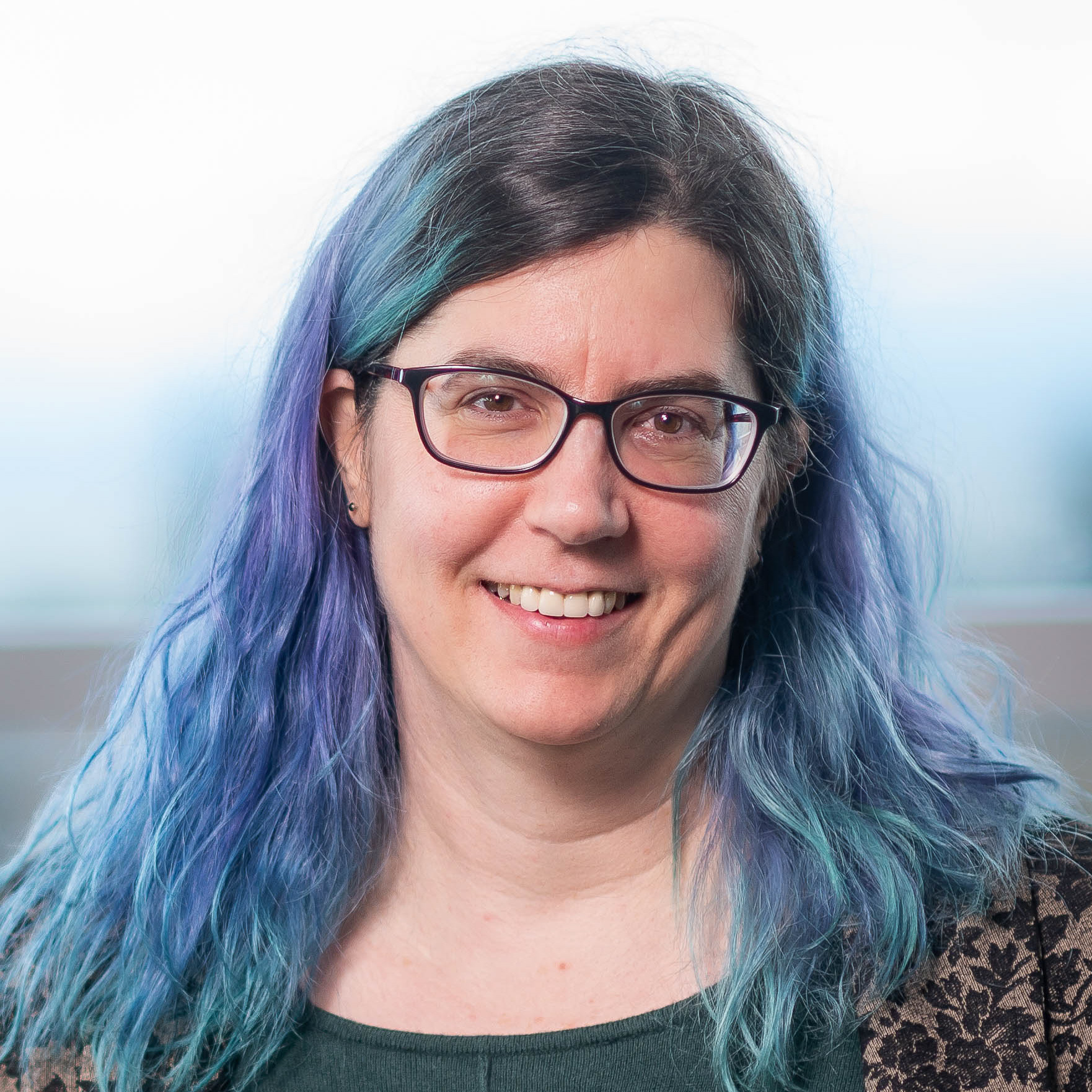
Tamara Munzner
Keynote: Reconnaissance and Recommendation: Answering Data Questions With Visualization
Tamara Munzner, University of British Columbia
Abstract: I'll frame visualization for data science through a set of metaphorical questions that are easy to state, but tricky to answer: Where are we? What's here? What's nearby? Are we there yet? Data reconnaissance and task wrangling is a conceptual framework for investigating where we are in complex and unfamiliar data landscape, through a four-phase cycle of acquire, view, assess, pursue. Recommendation through automatically generated layouts can shed light on that data landscape, and determining the right similarity measures is a way to operationalize the meaning of nearby. "Are we there yet?" is a question that's not just for road trips: it can apply to the training of a machine learning system.
Bio: Tamara Munzner is a Professor at the University of British Columbia Department of Computer Science, and holds a 2000 PhD from Stanford. She has been active in visualization research since 1991 and has published over ninety papers and chapters. She has been papers chair for IEEE InfoVis, EuroVis, and VIS, on the steering committees for InfoVis and BioVis, and the chair of the VIS Executive Committee. Her book Visualization Analysis and Design is widely used to teach visualization world-wide, and she is the co-editor of the A K Peters Visualization book series at CRC/Routledge. She received the IEEE VGTC Visualization Technical Achievement Award, multiple Test of Time Awards from InfoVis, and is an IEEE Fellow.
Closing