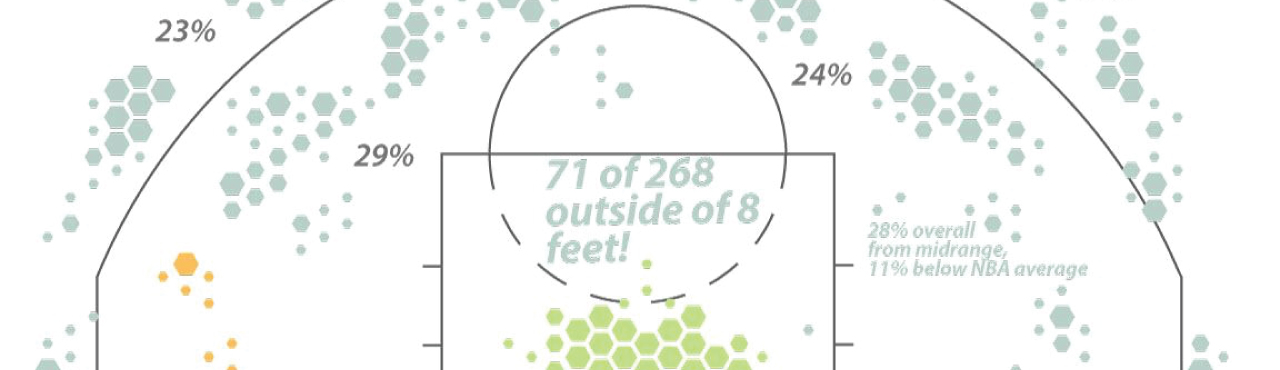
Program
VDS @IEEE VIS Program
Conference registration at http://ieeevis.org/year/2021/info/registration/conference-registration.
Sun. Oct 24, 2021, 8am - 12pm (US CENTRAL Time), 2021
Sun. 8:00am-9:10am (US CENTRAL Time)
Sun. 8:10am-9:10am
Opening & Keynote
Sun. 8:00am-8:10am (US CENTRAL Time)Opening
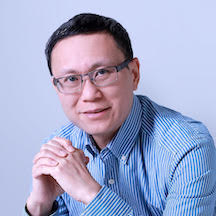
Jian Pei
Keynote: Towards Trustworthy Data Science - Challenges and Opportunities in Interpretability
Jian Pei, Simon Fraser University
Abstract: We believe data science and AI will change the world. No matter how smart and powerful AI models we can build, the ultimate testimony of the success of data science and AI is users' trust. How can we build trustworthy data science? At the level of user-model interaction, how can we convince users that a data analytic result is trustworthy? In this talk, I will brainstorm possible directions to the above questions in the context of an end-to-end data science pipeline. To strengthen trustworthy interactions between models and users, I will advocate exact and consistent interpretation of machine learning models. Our recent results show that exact and consistent interpretations are not just theoretically feasible, but also practical even for API-based AI services. Through reflection I will discuss some challenges and opportunities in building trustworthy data science for possible future work.
Bio: Jian Pei is a Professor in the School of Computing Science at Simon Fraser University. He is a renown leading researcher in the general areas of data science, big data, data mining, and database systems. His expertise is in developing effective and efficient data analysis techniques for novel data intensive applications, and transferring his research results to products and business practice. He is recognized as a Fellow of the Royal Society of Canada (Canada's national academy), the Canadian Academy of Engineering, the Association of Computing Machinery (ACM) and the Institute of Electrical and Electronics Engineers (IEEE). He is one of the most cited authors in data mining, database systems, and information retrieval. Since 2000, he has published one textbook, two monographs and over 300 research papers in refereed journals and conferences, which have been cited extensively by others. His research has generated remarkable impact substantially beyond academia. For example, his algorithms have been adopted by industry in production and popular open source software suites. Jian Pei also demonstrated outstanding professional leadership in many academic organizations and activities. He was the editor-in-chief of the IEEE Transactions of Knowledge and Data Engineering (TKDE) in 2013-16, the chair of the ACM Special Interest Group on Knowledge Discovery in Data (SIGKDD) in 2017-2021, and a general co-chair or program committee co-chair of many premier conferences. He maintains a wide spectrum of industry relations with both global and local industry partners. He is an active consultant and coach for industry. He received many prestigious awards, including the 2017 ACM SIGKDD Innovation Award, the 2015 ACM SIGKDD Service Award, the 2014 IEEE ICDM Research Contributions Award, the British Columbia Innovation Council 2005 Young Innovator Award, an NSERC 2008 Discovery Accelerator Supplements Award, an IBM Faculty Award (2006), a KDD Best Application Paper Award (2008), an ICDE Influential Paper Award (2018), a PAKDD Best Paper Award (2014), and a PAKDD Most Influential Paper Award (2009).
Sun. 9:10am-9:25am (US CENTRAL Time)
Break
Sun. 9:25am-10:25am (US CENTRAL Time)
Papers
Sun. 9:25am-9:40am (US CENTRAL Time)
Subhajit Das, Alex Endert
Abstract: Machine learning (ML) models are constructed by expert ML practitioners using various coding languages, in which they tune and select model hyperparameters and learning algorithms for a given problem domain. In multi-objective optimization, conflicting objectives and constraints is a major area of concern. In such problems, several competing objectives are seen for which no single optimal solution is found that satisfies all desired objectives simultaneously. In the past, visual analytic (VA) systems have allowed users to interactively construct objective functions for a classifier. In this paper, we extend this line of work by prototyping a technique to visualize multi-objective objective functions either defined in a Jupyter notebook or defined using an interactive visual interface to help users to detect and resolve conflicting objectives. Visualization of the objective function enlightens potentially conflicting objectives that obstructs selecting correct solution(s) for the desired ML task or goal. We also present an enumeration of potential conflicts in objective specification in multi-objective objective functions for classifier selection. Furthermore, we demonstrate our approach in a VA system that helps users in specifying meaningful objective functions to a classifier by detecting and resolving conflicting objectives.
Sun. 9:40am-9:55am (US CENTRAL Time)
Joseph Cottam, Maria Glenski, Zhuanyi Huang, Ryan Rabello, Austin Golding, Svitlana Volkova, Dustin L Arendt
Abstract: Reasoning about cause and effect is one of the frontiers for modern machine learning. Many causality techniques reason over a ``causal graph'' provided as input to the problem. When a causal graph cannot be produced from human expertise, ``causal discovery'' algorithms can be used to generate one from data. Unfortunately, causal discovery algorithms vary wildly in their results due to unrealistic data and modeling assumptions, so the results still need to be manually validated and adjusted. This paper presents a graph comparison tool designed to help analysts curate causal discovery results. This tool facilitates feedback loops whereby an analyst compares proposed graphs from multiple algorithms (or ensembles) and then uses insights from the comparison to refine parameters and inputs to the algorithms. We illustrate different types of comparisons and show how the interplay of causal discovery and graph comparison improves causal discovery.
Sun. 9:55am-10:10am (US CENTRAL Time)
Deepthi Raghunandan, Zhe Cui, Kartik Krishnan, Segen Tirfe, Shenzhi Shi, Tejaswi Darshan Shrestha, Leilani Battle, Niklas Elmqvist
Abstract: Keeping abreast of current trends, technologies, and best practices in visualization and data analysis is becoming increasingly difficult, especially for fledgling data scientists. In this paper, we propose Lodestar, an interactive computational notebook that allows users to quickly explore and construct new data science workflows by selecting from a list of automated analysis recommendations. We derive our recommendations from directed graphs of known analysis states, with two input sources: one manually curated from online data science tutorials, and another extracted through semi-automatic analysis of a corpus of over 6,000 Jupyter notebooks. We evaluate Lodestar in a formative study guiding our next set of improvements to the tool. The evaluation suggests that users find Lodestar useful for rapidly creating data science workflows.
Sun. 10:10am-10:25am (US CENTRAL Time)
Anamaria Crisan, Vidya Setlur
Abstract: Data analysts need to routinely transform data into a form conducive for deeper investigation. While there exists a myriad of tools to support this task on tabular data, few tools exist to support analysts with more complex data types. In this study, we investigate how analysts process and transform large sets of XML data to create an analytic data model useful to further their analysis. We conduct a set of formative interviews with four experts that have diverse yet specialized knowledge of a common dataset. From these interviews, we derive a set of goals, tasks, and design requirements for transforming XML data into an analytic data model. We implement Natto as a proof-of-concept prototype that actualizes these design requirements into a set of visual and interaction design choices. We demonstrate the utility of the system through the presentation of analysis scenarios using real-world data. Our research contributes novel insights into the unique challenges of transforming data that is both hierarchical and internally linked. Further, it extends the knowledge of the visualization community in the areas of data preparation and wrangling.
Sun. 10:25am-11:25am (US CENTRAL Time)
Sun. 10:25am-11:25am
Closing Keynote
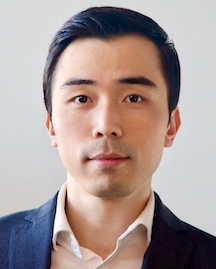
Polo Chau
Keynote: Towards Safe and Interpretable AI
Polo Chau, Georgia Tech
Abstract: Tremendous growth in artificial intelligence (AI) research has shown that AI is vulnerable to adversarial attacks, and their predictions can be difficult to understand, evaluate and ultimately act upon. Our Safe AI research thrust discovers real-world AI vulnerabilities and develops countermeasures to fortify AI deployment in safety-critical settings: ShapeShifter, the world's first targeted physical attack that fools faster R-CNN object detector; the UnMask defense that flags semantic incoherence in predictions (part of DARPA GARD); the TIGER toolbox for GPU-accelerated graph vulnerability and robustness analysis (part of Nvidia Data Science Teaching Kit); MalNet, the largest public cybersecurity graph database with over 1.2M graphs (100X more). Our complementary Interpretable AI research designs and develops interactive visualizations that amplify people’s ability to understand complex models and vulnerabilities, and provide key leaps of insight: Summit, NeuroCartography, and Bluff, systems that scalably summarize and visualize what features a deep learning model has learned, how those features interact to make predictions, and how they may be exploited by attacks; SkeletonVis, the first interactive tool that visualizes attacks on human action recognition models; CNN Explainer and GAN Lab (with Google Brain), accessible viral tools for students and experts to learn about AI models. We conclude by highlighting the next visual analytics research frontiers in AI.
Bio: Duen Horng (Polo) Chau is an Associate Professor of Computing at Georgia Tech. He co-directs Georgia Tech's MS Analytics program. He is the Director of Industry Relations of The Institute for Data Engineering and Science (IDEaS), and the Associate Director of Corporate Relations of The Center for Machine Learning. His research group bridges machine learning and visualization to synthesize scalable interactive tools for making sense of massive datasets, interpreting complex AI models, and solving real world problems in cybersecurity, human-centered AI, graph visualization and mining, and social good. His Ph.D. in Machine Learning from Carnegie Mellon University won CMU's Computer Science Dissertation Award, Honorable Mention. He received awards and grants from NSF, NIH, NASA, DARPA, Intel (Intel Outstanding Researcher), Google, Facebook, NVIDIA, Bosch, Amazon, Microsoft, Cisco, Symantec, eBay, Yahoo, LexisNexis; Raytheon Faculty Fellowship; Edenfield Faculty Fellowship; Outstanding Junior Faculty Award; The Lester Endowment Award; Symantec fellowship (twice); IEEE VIS'20 Best Poster Research Award, Honorable Mention; ACM TiiS 2018 Best Paper, Honorable Mention, Best student papers at SDM'14 and KDD'16 (runner-up); Best demo at SIGMOD'17 (runner-up); Chinese CHI'18 Best paper. His research led to open-sourced or deployed technologies by Intel (for ISTC-ARSA: ShapeShifter, SHIELD, ADAGIO, MLsploit), Google (GAN Lab), Facebook (ActiVis), Symantec (Polonium, AESOP protect 120M people from malware), and Atlanta Fire Rescue Department. His security and fraud detection research made headlines. He is a steering committee member of ACM IUI conference, IUI’15 co-chair, and IUI’19 program co-chair. He is an Associate Editor for IEEE TVCG. He was publicity chair for ACM KDD'14 and ACM WSDM'16 He co-organized the popular IDEA workshop (at KDD) that catalyzes cross-pollination across HCI and data mining.
Sun. 11:25am-11:30am (US CENTRAL Time)
Closing
KDD Program (Past)
Conference registration at https://kdd.org/kdd2021/attending.
Sun. August 15, 8am - 12pm (Singapore) / Sat. August 14, 5pm - 9pm (US West), 2021
Sun. 8:00am-9:10am (Singapore)/Sat. 5:00pm-6:10pm (US West)
Sun. 8:10am-9:10am (Singapore)/Sat. 5:10pm-6:10pm (US West)
Opening & Keynote
Sun. 8:00am-8:10am (Singapore)/Sat. 5:00pm-5:10pm (US West)Opening
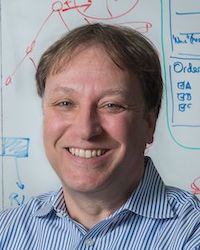
Hanspeter Pfister
Keynote: Towards Visually Interactive Neural Probabilistic Models
Hanspeter Pfister, Harvard University
Abstract: Deep learning methods have been a tremendously effective approach to problems in computer vision and natural language processing. However, these black-box models can be difficult to deploy in practice as they are known to make unpredictable mistakes that can be hard to analyze and correct. In this talk, I will present collaborative research to develop visually interactive interfaces for probabilistic deep learning models, with the goal of allowing users to examine and correct black-box models through visualizations and interactive inputs. Through co-design of models and visual interfaces, we will take the necessary next steps for model interpretability. Achieving this aim requires active investigation into developing new deep learning models and analysis techniques, and integrating them within interactive visualization frameworks.
Bio: Hanspeter Pfister is the An Wang Professor of Computer Science at the Harvard John A. Paulson School of Engineering and Applied Sciences and an affiliate faculty member of the Center for Brain Science. His research in visual computing lies at the intersection of visualization, computer graphics, and computer vision and spans a wide range of topics, including biomedical image analysis and visualization, image and video analysis, interpretable machine learning, and visual analytics in data science. Pfister has a PhD in computer science from the State University of New York at Stony Brook and an MS in electrical engineering from ETH Zurich, Switzerland. From 2013 to 2017 he was director of the Institute for Applied Computational Science. Before joining Harvard, he worked for over a decade at Mitsubishi Electric Research Laboratories, where he was associate director and senior research scientist. He was the chief architect of VolumePro, Mitsubishi Electric’s award-winning real-time volume rendering graphics card, for which he received the Mitsubishi Electric President’s Award in 2000. Pfister was elected as an ACM Fellow in 2019. He is the recipient of the 2010 IEEE Visualization Technical Achievement Award, the 2009 IEEE Meritorious Service Award, and the 2009 Petra T. Shattuck Excellence in Teaching Award. Pfister is a member of the ACM SIGGRAPH Academy, the IEEE Visualization Academy, and a director of the ACM SIGGRAPH Executive Committee and the IEEE Visualization and Graphics Technical Committee.
Sun. 9:10am-9:25am (Singapore)/Sat. 6:10pm-6:25pm (US West)
Break
Sun. 9:25am-10:25am (Singapore)/Sat. 6:25pm-7:25pm (US West)
Papers
Sun. 9:25am-9:40am (Singapore)/Sat. 6:25pm-6:40pm (US West)
Subhajit Das, Alex Endert
Abstract: Machine learning (ML) models are constructed by expert ML practitioners using various coding languages, in which they tune and select model hyperparameters and learning algorithms for a given problem domain. In multi-objective optimization, conflicting objectives and constraints is a major area of concern. In such problems, several competing objectives are seen for which no single optimal solution is found that satisfies all desired objectives simultaneously. In the past, visual analytic (VA) systems have allowed users to interactively construct objective functions for a classifier. In this paper, we extend this line of work by prototyping a technique to visualize multi-objective objective functions either defined in a Jupyter notebook or defined using an interactive visual interface to help users to detect and resolve conflicting objectives. Visualization of the objective function enlightens potentially conflicting objectives that obstructs selecting correct solution(s) for the desired ML task or goal. We also present an enumeration of potential conflicts in objective specification in multi-objective objective functions for classifier selection. Furthermore, we demonstrate our approach in a VA system that helps users in specifying meaningful objective functions to a classifier by detecting and resolving conflicting objectives.
Sun. 9:40am-9:55am (Singapore)/Sat. 6:40pm-6:55pm (US West)
Joseph Cottam, Maria Glenski, Zhuanyi Huang, Ryan Rabello, Austin Golding, Svitlana Volkova, Dustin L Arendt
Abstract: Reasoning about cause and effect is one of the frontiers for modern machine learning. Many causality techniques reason over a ``causal graph'' provided as input to the problem. When a causal graph cannot be produced from human expertise, ``causal discovery'' algorithms can be used to generate one from data. Unfortunately, causal discovery algorithms vary wildly in their results due to unrealistic data and modeling assumptions, so the results still need to be manually validated and adjusted. This paper presents a graph comparison tool designed to help analysts curate causal discovery results. This tool facilitates feedback loops whereby an analyst compares proposed graphs from multiple algorithms (or ensembles) and then uses insights from the comparison to refine parameters and inputs to the algorithms. We illustrate different types of comparisons and show how the interplay of causal discovery and graph comparison improves causal discovery.
Sun. 9:55am-10:10am (Singapore)/Sat. 6:55pm-7:10pm (US West)
Deepthi Raghunandan, Zhe Cui, Kartik Krishnan, Segen Tirfe, Shenzhi Shi, Tejaswi Darshan Shrestha, Leilani Battle, Niklas Elmqvist
Abstract: Keeping abreast of current trends, technologies, and best practices in visualization and data analysis is becoming increasingly difficult, especially for fledgling data scientists. In this paper, we propose Lodestar, an interactive computational notebook that allows users to quickly explore and construct new data science workflows by selecting from a list of automated analysis recommendations. We derive our recommendations from directed graphs of known analysis states, with two input sources: one manually curated from online data science tutorials, and another extracted through semi-automatic analysis of a corpus of over 6,000 Jupyter notebooks. We evaluate Lodestar in a formative study guiding our next set of improvements to the tool. The evaluation suggests that users find Lodestar useful for rapidly creating data science workflows.
Sun. 10:10am-10:25am (Singapore)/Sat. 7:10pm-7:25pm (US West)
Anamaria Crisan, Vidya Setlur
Abstract: Data analysts need to routinely transform data into a form conducive for deeper investigation. While there exists a myriad of tools to support this task on tabular data, few tools exist to support analysts with more complex data types. In this study, we investigate how analysts process and transform large sets of XML data to create an analytic data model useful to further their analysis. We conduct a set of formative interviews with four experts that have diverse yet specialized knowledge of a common dataset. From these interviews, we derive a set of goals, tasks, and design requirements for transforming XML data into an analytic data model. We implement Natto as a proof-of-concept prototype that actualizes these design requirements into a set of visual and interaction design choices. We demonstrate the utility of the system through the presentation of analysis scenarios using real-world data. Our research contributes novel insights into the unique challenges of transforming data that is both hierarchical and internally linked. Further, it extends the knowledge of the visualization community in the areas of data preparation and wrangling.
Sun. 10:25am-10:40am (Singapore)/Sat. 7:25pm-7:40pm (US West)
Break
Sun. 10:40am-11:40am (Singapore)/Sat. 7:40pm-8:40pm (US West)
Sun. 10:40am-11:40am (Singapore)/Sat. 7:40pm-8:40pm (US West)
Closing Keynote
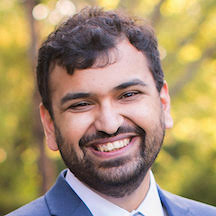
Arvind Satyanarayan
Keynote: From Tools to Toolkits - Towards more Reusable, Composable, and Reliable Machine Learning Interpretability
Arvind Satyanarayan, MIT
Abstract: As machine learning models are increasingly deployed into real-world contexts, the need for interpretability grows more urgent. In order to hold models accountable for the outcomes they produce, we cannot rely on quantitative measures of accuracy alone; rather, we also need to be able to qualitatively inspect how they operate. To meet this challenge, recent years have seen an explosion of research developing techniques and systems to interpret model behavior. But, are we making meaningful progress on this issue? In this talk, I will give us a language for answering this question by drawing on frameworks in human-computer interaction (HCI) and by analogizing to the progress of research in data visualization. I will use this language to characterize existing work (including work my research group is currently conducting) and sketch out directions for future work.
Bio: Arvind Satyanarayan is the NBX Assistant Professor of Computer Science in the MIT EECS department and a member of the Computer Science and Artificial Intelligence Lab (CSAIL). He leads the MIT Visualization Group which uses data visualization as a petri dish to study intelligence augmentation (IA), or how software systems can help amplify our cognition and creativity while respecting our agency. His work has been recognized with an NSF CAREER award and a Google Research Scholar award, best paper awards at premier academic venues (e.g., ACM CHI and IEEE VIS), and by practitioners (e.g., with an Information is Beautiful Award nomination). Visualization toolkits and systems he has developed with collaborators are widely used in industry (including at Apple, Google, and Microsoft), on Wikipedia, and in the Jupyter/Observable data science communities. Between 2018–2020, he served as a co-editor of Distill, an academic journal devoted to clarity in machine learning research.